Mit Daten gewinnen: Zusammenfassung und Rückblick
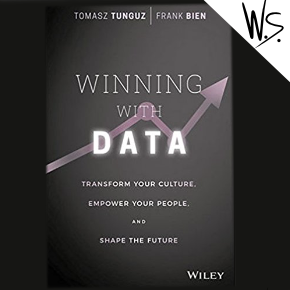
Schlüsselwörter: Analyse, Analytics, Business, Daten, Metriken, Informationen, Infrastruktur, Investoren, Operationalisieren, Start-up
Bitte beachten Sie: Am Ende dieses Beitrags finden Sie Links zu anderen Rezensionen, Zusammenfassungen und Ressourcen.
Buchrezension
In today’s world, data is changing every industry. Data is the future, and companies that understand how to use it and operationalize it have a huge advantage over those that do not. To succeed in this rapidly changing environment, everyone in a company should have immediate access to the information they need to make the best decisions. Mit Daten gewinnen berät, wie Unternehmen durch die strategische Analyse von Daten weiter wachsen und sich weiterentwickeln können.
The advent of cellphones has exponentially increased everyone’s need and desire for data. People expect data. They are used to having their questions answered immediately. In this data-rich environment, it’s important to avoid common biases and errors of perception. Within a company, data teams can be an important force for reducing bias and facilitating data literacy. The team should teach their colleagues to use data well; they should also help people learn to communicate about data more clearly.
Metrics can have a profound effect on process and reflect a company’s competitive edge, but that’s not the whole story. A company is more than its metrics. A company also needs well-defined values. And it needs the right people: people with intellectual honesty; people with curiosity; people who will use metrics to answer questions.
Die Co-Autoren von Mit Daten gewinnen both have backgrounds in data-heavy industries. Tomasz Tunguz is a venture capitalist at Redpoint Ventures, and his blog promotes data-driven advice for startups. Frank Bien — an outspoken believer in teamwork and positive corporate culture — is the CEO of Looker, a business intelligence platform. Those who are interested in learning about the authors’ careers and how they came to this collaboration are advised to read the introduction.
Tunguz and Bien use plenty of examples taken from their own experiences and those of other well-known companies: Venmo’s use of data to improve their products; Warby Parker’s disruption of a multi-billion-dollar market; ThredUp’s ability to process thousands of items a day.
Mit Daten gewinnen bietet Beratung, um Unternehmen zu helfen, sich in einer schönen neuen Welt zurechtzufinden. Die Empfehlungen der Autoren für die Gründung eines datengetriebenen Unternehmens werden Schritt für Schritt aufgeschlüsselt, einschließlich: Schaffung eines universellen Lexikons; Wiederbelebung der Teamkultur; Meetings auf Kurs halten; und hochwertige Präsentationen zu erstellen. Dieser nicht-technische Überblick erklärt hinreichend, wie die strategische Nutzung von Daten jedem Unternehmen einen Wettbewerbsvorteil verschaffen kann.
Zusammenfassung
Kapitel 1
Das Werbegeschäft hat früher die Art von kreativen Ansätzen bevorzugt, die in der TV-Show dargestellt werden Verrückte Männer. Heutzutage treibt die Mathematik die Strategie weit mehr an als die Kreativität. Anstatt von Verrückte Männer, Werbefachleute sind Math Men: Die Informationstechnologie bietet die Werkzeuge für die Entwicklung von Kampagnen; Algorithmen leiten die Entscheidungsfindung; Fast alle Arbeiten werden am Computer ausgeführt. Es hat sich einiges geändert seit dem Verrückte Männer Tage.
Daten haben eine Vielzahl unterschiedlicher Bereiche verändert, nicht nur die Werbung. Daten sind die Zukunft, und Unternehmen müssen verstehen, wie sie sie nutzen und sich mit ihnen weiterentwickeln.
In einem Unternehmen mit operationalisierten Daten bestimmen Daten das Verhalten jedes Mitarbeiters. Uber besitzt beispielsweise kein Inventar; das ganze Geschäft basiert auf Daten. Das Unternehmen entsendet Fahrer viel effizienter als altmodische Taxiunternehmen und sorgt durch ein Feedback-System, das problematische Fahrer leicht identifiziert, für eine hohe Zufriedenheit. Daten = operationalisiert.
Instant data has become crucial, and the demand for instantaneous information is growing. We want our questions answered immediately (!). Because it used to take too long for people to get information in their company, data has historically been used as a tool to gauge past performance. Companies with good data infrastructure, however, can produce information and make decisions based on current metrics. These companies can ensure that data can get to where it needs to be, and in front of those who need it, — instantly. Inefficient supply chains (the people, processes, and programs that touch the data) result in slow data, where more people are seeking it than supplying it. This was a problem back in the day, but today, we are data rich and there is always more to be harvested.
Die Datenmenge macht das Sortieren jedoch schwieriger und zeitaufwändiger als früher. Kleine Unternehmen haben möglicherweise keine Mitarbeiter für die Datenanalyse, und das Erstellen und Ausführen von Abfragen und Berichten kann überwältigend sein. Ohne Zugang zu adäquaten Daten gewöhnen sich Unternehmen daran, Entscheidungen auf der Grundlage von Meinungen zu treffen. Dies ist nie der beste Weg, um ein Unternehmen zu führen, und es kann ein Zeichen dafür sein, dass ein Unternehmen eine neue Datenlieferkette aufbauen muss.
Einige Unternehmen haben ganze Teams, die sich der Gewährleistung einer einheitlichen Art und Weise widmen, wie Daten gemessen, beschrieben und verwendet werden. Sie schulen andere im Unternehmen und befähigen sie, Daten kreativ zu nutzen. Datenteams demokratisieren den Datenzugriff, indem sie jedem helfen, besser zu verstehen, wie das Unternehmen mithilfe von Daten anstelle von Meinungen vorangebracht werden kann.
Kapitel 2
Es gibt einige Probleme mit Daten, die für unsere Zeit charakteristisch sind.
Etymologisch Neugierige werden sich für die Fleischmanns interessieren, tschechische Bäcker, die in die USA einwanderten und durch die heute noch in Supermärkten verkaufte Backhefe berühmt wurden. Die Fleischmanns machten jeden Tag Brot, und am Ende des Tages hatten sie immer etwas übrig, das sie an die Armen verschenkten. Die Schlangen von Menschen, die auf dieses kostenlose Brot warteten, wurden bekannt als Brotlinien. Heutzutage gibt es Brotzeiten für die Datenarmen. Die Leute warten auf die Informationen, die sie brauchen, wie ein armer Mensch auf Brot wartet. Einige Datenanfragen werden priorisiert; andere Anfragen warten. Daten-Breadlines verursachen mehrere Probleme:
- Die Leute müssen auf Daten warten. Dies verlangsamt den Entscheidungsprozess, was wiederum das Unternehmen verlangsamt.
- Die Leute werden ungeduldig und treffen manchmal Entscheidungen, ohne auf Daten zu warten. Uninformierte Vermutungen führen selten zu guten Ergebnissen.
- Die Einhaltung der Brotgrenze entzieht dem Datenmanagement-Team Energie, behindert ihr Potenzial und vergeudet ihre Talente.
Auch die Datenunklarheit ist problematisch. Reaktionszeit und Genauigkeit verlangsamen sich, wenn Daten desorganisiert sind. Schließlich kann ein Unternehmen das Vertrauen in seine Daten verlieren.
Data fragmentation is another problem. When people can’t get the data they need, they find a way to capture it and create their own databases. Rogue analysts and shadow databases often ignore normal validation and updating processes, keeping the information in silos.
Finally, data brawls create significant issues for companies. Data segmentation can create areas of misalignment. If there isn’t consistency in information, people start mistrusting each other’s point of view. They disagree; they argue; they fight. People in companies all need to be on the same page. They have to be using the same metrics and the same lexicon.
Kapitel 3
Business-Intelligence-Systeme bestehen traditionell aus drei Schichten: Eine Datenbank speichert die Daten; ein Data Warehouse sammelt die Daten aus der Datenbank und aggregiert sie; und eine Visualisierungsschicht formatiert und präsentiert Berichte für den Endbenutzer. Dies ist eine Art knarrendes altes System, bei dem jedes Mal neue Abfragen für neue Berichte geschrieben werden müssen, wenn eine andere Frage gestellt wird.
Back when they were a little startup, Google had vast amounts of data, but they couldn’t afford Oracle’s database fees. To get around this problem, they bought their own servers and distributed their data among them. The strategy worked, and, as I’m sure you are aware, Google is a model of data management today. The company has generated obscene amounts of data, and Google employees use this data for all kinds of research and analysis.
Data analysis is also taken seriously at Facebook, which has developed a number of different technologies to provide employees with access to data. One interface, HiPal, makes it easier for analysts to search for data. Users who aren’t familiar with SQL (Structured Query Language (SQL)) can do the same kind of analyses using these company technologies as one can with SQL. Other companies, like LinkedIn, use a similar data infrastructure.
Looker ist eine neue Art von Datenschnittstelle. Es erstellt eine einzige Version von allem, die von der gesamten Organisation verwendet werden kann, wodurch die Datenintegrität erheblich verbessert wird.
Extreme Datensammlung ist die neue Normalität; alle großen Unternehmen verfügen über diese leistungsstarken Datenbanken. Sie sind sehr schnell, die Lagerung ist billig und es gibt viel Platz und Möglichkeiten. Angesichts dieser Fortschritte muss der gesamte Analyseansatz aktualisiert werden. Riesige Informationsmengen können angehäuft werden, und versierte Mitarbeiter sind es gewohnt, auf Daten zuzugreifen. Sie benötigen ausgereifte Tools, um anspruchsvolle Informationsbedürfnisse zu erfüllen. Und je einfacher es ist, die Tools zu verwenden, desto mehr Menschen werden sie verwenden.
Heutzutage gibt es viele Daten zu erforschen und die Menschen haben die Freiheit, sie zu erkunden. Dies ist die Datenstruktur der modernen Welt.
Eine kurze Lektion zur Geschichte der Datentechnologie: Die Datenbank wurde 1970 von einem IBM-Mitarbeiter namens Edgar Cook erfunden. Oracle Systems wurde zum dominierenden Entwickler von Datenbanken und verdiente viel Geld mit der Speicherung von Daten in ihren Datenbanken. In den 1990er Jahren führten andere Unternehmen Software ein, die die Nutzung von Datenbanken vereinfachte und die Datenbankkosten minimierte.
Kapitel 4
In der Regel verwenden Unternehmen Daten, um zu untersuchen, was in der Vergangenheit passiert ist. Der neue Weg besteht darin, Daten zu operationalisieren und sie zu verwenden, um Ereignisse zu verstehen, sobald sie auftreten.
Back in the day, clothes and fabric were expensive enough that even aristocrats bought used clothes. People called the Strazzaroli dealt in high-end used clothes. But as the industrial revolution ramped up, clothes got cheaper, and the Strazzaroli lost their means of living. Fast forward to a modern consignment company, The RealReal. They use real-time reporting to see what’s in their warehouse and how everything is moving in the value chain. Everyone in the company has access to the same information; everyone can react to the data in real-time. Design, marketing, finance, operations — e everyone can use instant information to benefit the company.
ThredUp ist ein weiterer Händler für gebrauchte Kleidung. Neben der Verfolgung und Verarbeitung von Waren verwendet ThredUp Daten, um vorherzusagen, welche Arten von Kleidung zu einem bestimmten Zeitpunkt nachgefragt werden. Die Verwaltung ihrer Daten half ihnen, nach der Einführung schnell zu skalieren.
Companies spend too much time on trivialities. Meetings eat up everyone’s time. This is lost productivity. The right data, however, reduces meeting time because it helps people focus on the right questions.
HubSpot, Anbieter von Marketing-Automatisierungssoftware, verfolgt fünf Metriken, um die Leistung seiner Vertriebsmitarbeiter zu bewerten. Vertriebsmitarbeiter können auf ihr eigenes Dashboard zugreifen, um zu sehen, wie sie ihre Ziele erreichen. Looker, wie bereits erwähnt, hat auch ein Tool entwickelt, um die Verkaufsleistung zu verfolgen. Vertriebsmitarbeiter können sehen, wie nahe sie ihrem Kontingent sind und was sie in der Pipeline haben. Zendesk, Anbieter von Kundenservicelösungen, nutzt NPS-Kundenumfragen, um Daten zu generieren, die ihnen zu einem beeindruckenden Wachstum verholfen haben.
Daten sind ein wichtiger Bestandteil jedes erfolgreichen modernen Unternehmens. Es spielt eine wichtige Rolle bei der Vermarktung von Lagerbeständen, der Reaktion auf Kundenanfragen, dem rechtzeitigen Aufbau von Vertriebsmitarbeitern und der Erhöhung der Reaktionsgeschwindigkeit.
Dieses Kapitel ist reich an Ratschlägen der Autoren:
- It’s important to have the same metrics across the company. Consider formalizing and standardizing using something akin to a data dictionary. You need to have a common lexicon.
- Be brutally honest — or at least aim for that ideal. People shouldn’t be sensitive. Let go of your ego; accept criticism.
Decision making can be really arbitrary if it’s not backed up with data. The more information we have, the better decisions we make.
Kapitel 5
Curiosity is a basic human emotion and, according to the authors, the best way to transform a company’s culture into one that is data driven. Employees should be curious. They should have the ability to look up the information in which they are interested, and they should be able to test their hypotheses.
Wenn ein Unternehmen von Daten angetrieben wird, sind einige kulturelle Veränderungen zu erwarten:
- Das Unternehmen beginnt, Daten zu verwenden, um Entscheidungen zu treffen.
- Die besten Ideen bekommt das Unternehmen von allen, nicht nur von den Führungskräften.
- Das Unternehmen fördert Experimente und Überraschungen.
Experimenting is important. Demonstrating the value of experimentation, the authors discuss Intuit’s payroll management product, Paycycle. Product managers thought about putting in a feature enabling employers to cut checks immediately, but research indicated clients wouldn’t be interested in such a feature. They decided to test the feature anyway, and it ended up being surprisingly popular. The right culture starts with employees who are curious; it starts with people who ask questions.
Finding curious people is important, and it starts with the hiring process. But hiring interviews aren’t usually very informative. They can be rather haphazard. Instead, the authors suggest there should be a systematic process which could include determining desirable qualities in a candidate, crafting interview questions that address these qualities, and scoring candidates on the desired attributes. The candidate with the best score wins.
Recruiting metrics are useful to evaluate hiring practices — for example, the number of qualified candidates who pass a phone interview, the time from first contact with a candidate to signed offer, etc. To monitor satisfaction, you can survey candidates after interviews to see what they thought of the experience. Another important metric is the offer-acceptance rate (the percentage of people who accept job offers). Calculate your hires to goal by dividing the number of hires by the hiring goal.
At the end of the day, you want employees who will fit the company culture. But how do you measure culture? Use surveys and other tools to establish a dialogue between management and employees about the company. What are people’s goals? What do they like about the company? What feedback can they give? This process continues until the company values are crystallized and can be recorded.
Clarifying these pieces will make it easier for the interviewer to determine the extent to which a candidate’s values are a good fit. For example, if your company values high-quality customer service, you might ask an employee for an example of a time they helped a client.
Google geht mit Metriken einen Schritt weiter als alle anderen. Sie messen absolut alles über den Einstellungsprozess und geben ihren HR-Mitarbeitern viel Feedback. Interviewer erhalten routinemäßig Informationen, um ihre Leistung zu verbessern.
Kapitel 6
Once you have curious employees, expect that they’ll be asking questions, which starts the typical progression in data-driven companies:
- Schritt eins: Personen, die Informationen benötigen, fragen einen der Ingenieure, die bei der Erstellung und Erstellung der Datensysteme mitgewirkt haben. Wenn das Unternehmen wächst, wird dies zu einer Belastung für die Ingenieure.
- Step two: The team borrows a solution from somewhere else. People use software or other tools from another department or another company. Tailored for someone else’s data, this might not be a good fit.
- Schritt drei: Das Team erhält die Rohdaten und schreibt eigene Abfragen.
Twilio hatte zwei Arten von Datensuchern. Einerseits wusste das Datenteam alles über die Dateninfrastruktur und deren Nutzung. Sie wünschten sich Berichte, die mehrfach ausgeführt und an das entsprechende Publikum geliefert werden konnten. Auf der anderen Seite wollte der Rest des Unternehmens eine einfache Schnittstelle, die es ihnen ermöglicht, durch die Daten zu blättern. Die Befriedigung dieser beiden sehr unterschiedlichen Zielgruppen ist eine kritische Aufgabe der Dateninfrastruktur.
Problematisch ist auch die Dezentralisierung der IT-Einkaufskompetenz. Teamleiter und Abteilungen kaufen zunehmend Software, wodurch das Datenteam aus der Schleife herausgeschnitten wird. (Diese fehlende Rechenschaftspflicht gegenüber der technischen Abteilung wird Schatten-IT genannt.) Anbieter sind gerne bereit, ihren leitenden Kunden maßgeschneiderte Lösungen anzubieten, aber dies führt zu einer Datenfragmentierung, bei der verschiedene Abteilungen und Einheiten unterschiedliche Versionen der Wahrheit haben.
Datenteams müssen die Datenarchitektur transformieren, um den Benutzern mehr Macht zu geben. Endbenutzer sollten entscheiden, welche Berichtstools sie verwenden möchten. Die Rolle des Datenteams besteht darin, die Infrastruktur zu unterstützen, damit Benutzer die Daten analysieren können. Cloud-Datenbanken sollten mit lokalen Unternehmensdatenbanken funktionieren.
The data fabric — the matrix of information within the company — must be accessible to everyone, and one way to standardize it is through data modeling. Everyone in the company should use the same numbers and speak the same language. Consistency is so important. Scientists and engineers might understand the data architecture of a company, but not everyone else will. Data fabric makes the information available to all.
Kapitel 7
During World War II, a group of mathematicians and statisticians had secret meetings in New York where they analyzed military data and made recommendations to Washington (which were frequently followed). One of the guys on the team, Abraham Wald, had been asked by the Air Force to design armor for airplanes. Data from returning planes showed that most of the bullet holes were located around the tail gunner and the wings, so people thought these were the areas that should wear the armor. (The armor was heavy so they couldn’t just slap it on the whole plane. They had to be selective.) But Abraham Wald pointed out that the planes that had been shot in the wings were the planes that lived to tell the tale. The authors tell this story to illustrate the importance of avoiding data bias.
Es gibt viele potenzielle Fallstricke, viele Arten von Datenverzerrungen, die Sie daran hindern können, Daten zu verstehen:
- Survivorship bias — Any time you cut data from your analysis, you risk distorted results. Correlation is not causation; just because two things seem to go together doesn’t mean that the one caused the other.
- Anchoring bias — This occurs when someone suggests a value to you and it affects your own estimate. For example, if I ask whether Gandhi was over 114 when he died, your answer would probably be different than if I asked whether he was over 35.
- Availability bias — If you see something happen or hear about how it happened from someone you know, it will seem like a lot more common an occurrence.
Sie können sich Illusionen der Validität machen und glauben, dass das Sammeln von mehr Daten dazu beiträgt, die Zukunft vorherzusagen, aber es gibt viele Möglichkeiten, wie Sie die Daten nicht richtig interpretieren können. Vorsichtig sein.
Neue Facebook-Mitarbeiter nehmen an einem zweiwöchigen Datencamp teil, um mehr Datenkompetenz zu erlangen. Dies gibt allen einen gemeinsamen Hintergrund, um Probleme und Chancen zu diskutieren. Sie lernen verfügbare Tools und Datensätze kennen. Außerdem haben sie die Möglichkeit, an Projekten mitzuarbeiten, um ihr Wissen zu erweitern. Datenteams können viel tun, um sich mit Mitarbeitern zu treffen und die Datenkompetenz im gesamten Unternehmen zu verbessern. Eine solche Auseinandersetzung mit der Unternehmenskultur ist ein wichtiger Bestandteil ihrer Arbeit.
Kapitel 8
Deskriptive Analysen fragen, was passiert ist; Die diagnostische Analytik fragt nach dem Warum. (Dashboards, die in diesem Buch irgendwie verleumdet werden, sind die Schnittstellen der deskriptiven Analyse.)
Descriptive and diagnostic analytics look at the past, while predictive and prescriptive analytics are about the future. Predictive analytics use historical data to predict future outcomes. Analysts can pose hypothetical “what if” questions to decide which path to take. Prescriptive analytics recommend the course of action based on the data. This requires lots of data and sophisticated analytics.
The Data Sophistication Journey is a model developed by Gartner, a marketing research agency. Data Sophistication maps a team’s evolution from descriptive to diagnostic analytics and from predictive to prescriptive analytics. But Gartner misses something between diagnostic and predictive analytics: exploratory analytics. This helps us find a hypothesis; this asks “why?” Confirmative analytics is used to determine if a hypothesis is true.
Data is only useful if you can act on it. Collecting data for no real reason serves no real purpose. On the other hand, you don’t always know what metrics will be actionable until after you’ve done an analysis. It’s good to have a balance.
Certain metrics are tried and true. The lifetime value of a customer (LTV) is an estimate of the total gross profit to be made from a customer over time. The cost of customer acquisition (CAC) is the total of all sales and marketing expenses averaged for one customer. The LTV/ CAC ratio indicates how efficiently a company pulls in revenue. But sometimes new metrics can be tailored to fit a situation, and creating new metrics can uncover new opportunities. The mMedia site Upworthy tracks various metrics to assess which factors make their content more popular, but they needed more information, so they invented a whole new metric to measure actual user attention (i.e., not accounting for those moments in which a web page open but the reader went to feed the cat). .
Design an experiment. Determine actionability. The data should relate to actual decisions that can be made. Bookend the expected results. Determine ahead of time the parameters of the experiment. Design the experiment. Develop a hypothesis. Decide on several different data points. Calculate the p-value. The p-value is the probability that the hypothesis is incorrect. Instructions for this calculation are provided. Plan to run the experiment. Figure out how long it will take. How many samples you need. Who will do the work, and how it will be structured. Don’t forget to include a control group to check results against. Run the experiment. Analyze the results. Compare them to the control group.
Kapitel 9
New York City hat der Öffentlichkeit eine Reihe von Daten zur Verfügung gestellt. Ein Stipendiat, Ben Wellington, begann mit der Analyse der Daten und berichtete in seinem Blog darüber, unter anderem über die Kartierung von Fahrradunfällen in der Stadt. Er wurde sehr populär und schreibt seinen Erfolg seinen Fähigkeiten im Geschichtenerzählen zu.
Wellington’s lessons include the importance of making data relatable. Turn it into stories. (I tell my team this all the time!) Some people think data is kind of boring, and it isn’t enough by itself to inspire them. By making it a story, you give it emotional appeal. It is particularly important to be able to tell your story when you’re courting investors. Entrepreneurs pitching startups to investors need to show that they have identified a new opportunity — demonstrate urgency.
A standard method of communicating data is through presentations. Start by defining the goal of the presentation. What are you trying to explain? Are you trying to convince someone of something? Are you trying to sell something? Evaluate the intended audience. Investors are particularly interested in risk, so discussing these points shows that you understand the investor’s perspective. (There are many kinds of risk, see sidebar.) It’s important to develop the story arc. With your knowledge of the investors’ hopes and worries, create a storyline that addresses those concerns. Aim to keep it to ten 10 slides or less.
The presentation could begin with the company purpose or mission. Describe the problem. What is wrong that your product will solve? Then offer a solution to the problem. Explain what makes this a good idea right now, and why someone didn’t do it before. A demonstration of your product would be nice, but even pictures are good. Other important information to include in your presentation: market size, your team, business model, the competition, and financials.
Communicate your vision of the opportunity, and reinforce the vision with data. Provide a solution. Explain the company’s approach. Demonstrate how the market has responded. Engagement and acquisition metrics will be helpful here. It’s important to offer a good estimate of the market size. Venture capitalists totally want to know about this. How big of a potential market are we talking here? A discussion of the financials should at minimum include revenue, gross margin, and cash flow.
When you give the presentation, people will have questions. The more data you have, the better prepared you’ll be to answer those questions.
Einige verschiedene Arten von Risiken:
Market timing risk — Is this the right time for this enterprise?
Business model risk — Do you have the right model for your product?
Market adoption risk — Will people use your new product?
Market size risk — Is your solution big enough to make a venture capitalist happy?
Execution risk — Does your team have the right skills for the job?
Technology risk — If new technology is developed, will it be finished on schedule?
Capitalization risk — Is there enough capital to go the distance?
Platform risk — Are there external partners outside your control?
Venture management risk — Is the company open to feedback?
Financial risk — Can the company keep paying the bills?
Legal risk — Are lawsuits or other legal issues looming on the horizon?
Kapitel 10: Alles zusammenfügen
Alles zusammenfügen
In einem Unternehmen kann es viele Reibungen geben. Daten können helfen, diese Reibung zu verringern.
People need to understand and expect data. People need to be intellectually honest; the decision-making process isn’t about ego. Let the best ideas win and don’t let politics affect the choice. A company needs well defined values. It needs the right people, it needs curious people.
Der beste Weg, ein Unternehmen für diese schöne neue Welt zu wappnen, sind Daten, und der Durst nach Daten ist zunehmend global. Es verändert wirklich jede Branche. In Zukunft wird die Operationalisierung von Daten Unternehmen den Wettbewerbsvorteil verschaffen, den sie für ihren Erfolg benötigen. Die Menschen haben sofort die Informationen, die sie benötigen, um die besten Entscheidungen zu treffen.
Metriken können einen tiefgreifenden Einfluss auf den Prozess haben. Sie können die Arbeitsweise eines Unternehmens verbessern, was ihm einen Wettbewerbsvorteil verschaffen kann. Mit einer einheitlichen Datenstruktur und guten Teams transformieren Unternehmen ihre Branchen.
Zusätzliche Ressourcen
Bewertung von Mit Daten gewinnen von Sloan Review
Rezension zu Mit Daten gewinnen von Hacker Noon
Rezension zu Mit Daten gewinnen von Kelly Sutton
Interview mit dem Autor von Winning With Data by Mix Panel
Kaufen Sie mit Daten gewinnen bei Amazon
Zusammenfassung des Gewinnens mit Daten durch umsetzbare Bücher Kostenlos
Zusammenfassung des Gewinnens mit Daten von Get Abstract Freemium