Winning With Data: Summary and Review
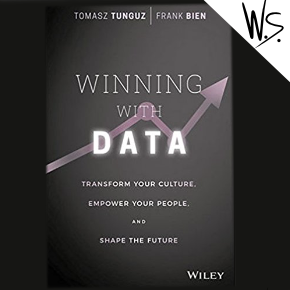
Keywords: Analysis, Analytics, Business, Data, Metrics, Information, Infrastructure, Investors, Operationalize, Start-up
Please Note: There are links to other reviews, summaries and resources at the end of this post.
Book Review
In today’s world, data is changing every industry. Data is the future, and companies that understand how to use it and operationalize it have a huge advantage over those that do not. To succeed in this rapidly changing environment, everyone in a company should have immediate access to the information they need to make the best decisions. Winning with Data offers advice on how companies can continue to grow and evolve through the strategic analysis of data.
The advent of cellphones has exponentially increased everyone’s need and desire for data. People expect data. They are used to having their questions answered immediately. In this data-rich environment, it’s important to avoid common biases and errors of perception. Within a company, data teams can be an important force for reducing bias and facilitating data literacy. The team should teach their colleagues to use data well; they should also help people learn to communicate about data more clearly.
Metrics can have a profound effect on process and reflect a company’s competitive edge, but that’s not the whole story. A company is more than its metrics. A company also needs well-defined values. And it needs the right people: people with intellectual honesty; people with curiosity; people who will use metrics to answer questions.
The co-authors of Winning with Data both have backgrounds in data-heavy industries. Tomasz Tunguz is a venture capitalist at Redpoint Ventures, and his blog promotes data-driven advice for startups. Frank Bien — an outspoken believer in teamwork and positive corporate culture — is the CEO of Looker, a business intelligence platform. Those who are interested in learning about the authors’ careers and how they came to this collaboration are advised to read the introduction.
Tunguz and Bien use plenty of examples taken from their own experiences and those of other well-known companies: Venmo’s use of data to improve their products; Warby Parker’s disruption of a multi-billion-dollar market; ThredUp’s ability to process thousands of items a day.
Winning with Data offers advice to help companies navigate a brave new world. Author recommendations for creating a Data-Driven Company are broken down step by step, including: creating a universal lexicon;, revitalizing team culture; keeping meetings on track; and making quality presentations. This non-technical overview adequately explains how the strategic use of data can give any company a competitive advantage.
Summary
Chapter 1
The advertising business used to favor the sort of creative approaches depicted on the TV show Mad Men. These days, math drives strategy far more than creativity. Instead of Mad Men, advertising professionals are Math Men: Information Technology provides the tools for developing campaigns; algorithms guide decision-making; nearly all work is performed on computers. Quite a bit has changed since the Mad Men days.
Data has transformed a wide range of diverse fields, not just advertising. Data is the future, and companies must understand how to use it and evolve with it.
In a company that has operationalized data, data drives the behavior of every employee. For example, Uber owns no inventory; the whole business is based on data. The company dispatches drivers much more efficiently than old-fashioned taxi companies do, and it maintains high satisfaction through a feedback system that easily identifies problem drivers. Data = operationalized.
Instant data has become crucial, and the demand for instantaneous information is growing. We want our questions answered immediately (!). Because it used to take too long for people to get information in their company, data has historically been used as a tool to gauge past performance. Companies with good data infrastructure, however, can produce information and make decisions based on current metrics. These companies can ensure that data can get to where it needs to be, and in front of those who need it, — instantly. Inefficient supply chains (the people, processes, and programs that touch the data) result in slow data, where more people are seeking it than supplying it. This was a problem back in the day, but today, we are data rich and there is always more to be harvested.
The volume of data, however, makes sorting through it more difficult and time consuming than it used to be. Small companies might not have data analytics people, and creating and running queries and reports can become overwhelming. Without access to adequate data, companies get used to making decisions based on opinion. This is never the best way to run a business, and it might be sign that a company needs to build a new data supply chain.
Some businesses have entire teams devoted to ensuring uniformity in how data is measured, described, and used. They tutor others in the company and empower them to use data creatively. Data teams democratize data access by helping everyone better understand how to advance the company using data instead of opinions.
Chapter 2
There are some problems with data that are characteristic of our age.
The etymologically curious will be interested in learning about the Fleischmanns, Czech bakers who immigrated to the United States and became famous for the baking yeast still sold in supermarkets today. The Fleischmanns made bread every day, and they always had some left over at the end of the day, which they gave away to the poor. The lines of people waiting for this free bread became known as breadlines. Today, there are breadlines for the data poor. People wait for the information they need like a poor person waits for bread. Some data requests are prioritized; other requests are left waiting. Data breadlines cause multiple problems:
- People have to wait for data. This slows down the decision-making process, which, in turn, slows down the company.
- People get impatient, sometimes making decisions without waiting for data. Uninformed guesswork rarely leads to good results.
- Minding the breadline drains energy from the data management team, hinders their potential, and squanders their talents.
Data obscurity is also problematic. Response time and accuracy slows when data is disorganized. Eventually, a company can lose confidence in their data.
Data fragmentation is another problem. When people can’t get the data they need, they find a way to capture it and create their own databases. Rogue analysts and shadow databases often ignore normal validation and updating processes, keeping the information in silos.
Finally, data brawls create significant issues for companies. Data segmentation can create areas of misalignment. If there isn’t consistency in information, people start mistrusting each other’s point of view. They disagree; they argue; they fight. People in companies all need to be on the same page. They have to be using the same metrics and the same lexicon.
Chapter 3
Business intelligence systems traditionally have three layers: a database stores the data; a data warehouse gathers the data from the database and aggregates it; and a visualizing layer formats and presents reports for the end user. This is kind of a creaky old system in that new queries must be written for new reports every time a different question is asked.
Back when they were a little startup, Google had vast amounts of data, but they couldn’t afford Oracle’s database fees. To get around this problem, they bought their own servers and distributed their data among them. The strategy worked, and, as I’m sure you are aware, Google is a model of data management today. The company has generated obscene amounts of data, and Google employees use this data for all kinds of research and analysis.
Data analysis is also taken seriously at Facebook, which has developed a number of different technologies to provide employees with access to data. One interface, HiPal, makes it easier for analysts to search for data. Users who aren’t familiar with SQL (Structured Query Language (SQL)) can do the same kind of analyses using these company technologies as one can with SQL. Other companies, like LinkedIn, use a similar data infrastructure.
Looker is a new kind of data interface. It creates a single version of everything to be used by the whole organization, significantly improving data integrity.
Extreme data collection is the new normal; all the big companies have these high-performance databases. They are very fast, storage is cheap, and there is plenty of space and capability. Given these advances, the whole approach to analytics needs to be updated. Vast amounts of information can be amassed, and savvy workers are used to having access to data. They need sophisticated tools to meet sophisticated information needs. And the easier it is to use the tools, the more people will use them.
These days, there is a lot of data to explore and people have the freedom to explore it. This is the data fabric of the modern world.
A quick lesson on the history of data technology: The database was invented in 1970 by an IBM employee named Edgar Cook. Oracle Systems became the dominant developer of databases and made lots of money storing data in their databases. In the 1990s, other companies introduced software that made it easier to use databases and minimized database expenses.
Chapter 4
Typically, companies use data to look at what happened in the past. The new way is to operationalize data and use it to understand events as they occur.
Back in the day, clothes and fabric were expensive enough that even aristocrats bought used clothes. People called the Strazzaroli dealt in high-end used clothes. But as the industrial revolution ramped up, clothes got cheaper, and the Strazzaroli lost their means of living. Fast forward to a modern consignment company, The RealReal. They use real-time reporting to see what’s in their warehouse and how everything is moving in the value chain. Everyone in the company has access to the same information; everyone can react to the data in real-time. Design, marketing, finance, operations — e everyone can use instant information to benefit the company.
ThredUp is another used-clothing dealer. In addition to tracking and processing merchandise, ThredUp uses data to predict what kinds of clothes will be in demand at any given time. Managing their data helped them scale quickly after they launched.
Companies spend too much time on trivialities. Meetings eat up everyone’s time. This is lost productivity. The right data, however, reduces meeting time because it helps people focus on the right questions.
HubSpot, providers of marketing automation software, tracks five metrics to evaluate the performance of their sales staff. Sales staff can access their own dashboard to see how they are progressing toward their goals. Looker, discussed earlier, also created a tool to track sales performance. Sales staff can see how close they are to meeting their quota, as well as monitor what they have in the pipeline. Zendesk, providers of customer service solutions, use NPS customer surveys to generate data, which has helped them sustain impressive growth.
Data is an important part of any successful modern business. It plays an important role in merchandising inventory, responding to customer requests, ramping up salesforces at the right time, and increasing reaction speed.
This chapter is rich in advice from the authors:
- It’s important to have the same metrics across the company. Consider formalizing and standardizing using something akin to a data dictionary. You need to have a common lexicon.
- Be brutally honest — or at least aim for that ideal. People shouldn’t be sensitive. Let go of your ego; accept criticism.
Decision making can be really arbitrary if it’s not backed up with data. The more information we have, the better decisions we make.
Chapter 5
Curiosity is a basic human emotion and, according to the authors, the best way to transform a company’s culture into one that is data driven. Employees should be curious. They should have the ability to look up the information in which they are interested, and they should be able to test their hypotheses.
When a company becomes driven by data, there are a few cultural shifts that can be expected:
- The company starts using data to make decisions.
- The company gets the best ideas from everyone, not just the executives.
- The company encourages experimentation and surprises.
Experimenting is important. Demonstrating the value of experimentation, the authors discuss Intuit’s payroll management product, Paycycle. Product managers thought about putting in a feature enabling employers to cut checks immediately, but research indicated clients wouldn’t be interested in such a feature. They decided to test the feature anyway, and it ended up being surprisingly popular. The right culture starts with employees who are curious; it starts with people who ask questions.
Finding curious people is important, and it starts with the hiring process. But hiring interviews aren’t usually very informative. They can be rather haphazard. Instead, the authors suggest there should be a systematic process which could include determining desirable qualities in a candidate, crafting interview questions that address these qualities, and scoring candidates on the desired attributes. The candidate with the best score wins.
Recruiting metrics are useful to evaluate hiring practices — for example, the number of qualified candidates who pass a phone interview, the time from first contact with a candidate to signed offer, etc. To monitor satisfaction, you can survey candidates after interviews to see what they thought of the experience. Another important metric is the offer-acceptance rate (the percentage of people who accept job offers). Calculate your hires to goal by dividing the number of hires by the hiring goal.
At the end of the day, you want employees who will fit the company culture. But how do you measure culture? Use surveys and other tools to establish a dialogue between management and employees about the company. What are people’s goals? What do they like about the company? What feedback can they give? This process continues until the company values are crystallized and can be recorded.
Clarifying these pieces will make it easier for the interviewer to determine the extent to which a candidate’s values are a good fit. For example, if your company values high-quality customer service, you might ask an employee for an example of a time they helped a client.
Google takes metrics one step further than everyone else. They measure absolutely everything about the hiring process, and they give their HR people lots of feedback. Interviewers routinely receive information to improve their performance.
Chapter 6
Once you have curious employees, expect that they’ll be asking questions, which starts the typical progression in data-driven companies:
- Step one: People who need information ask one of the engineers who helped create and build the data systems. As the company grows, this becomes a burden on the engineers.
- Step two: The team borrows a solution from somewhere else. People use software or other tools from another department or another company. Tailored for someone else’s data, this might not be a good fit.
- Step three: The team gets the raw data and writes their own queries.
Twilio had two kinds of data seekers. On one hand, the data team knew everything about the data infrastructure and how to use it. They liked to have reports that could be run numerous times and delivered to the appropriate audience. On the other hand, the rest of the company wanted a simple interface that would allow them to browse through the data. Satisfying these two very different constituencies is a critical mission of data infrastructure.
Also problematic is the decentralization of IT purchasing authority. Team leaders and departments are increasingly purchasing software, cutting the data team out of the loop. (This lack of accountability to the tech department is called Shadow IT.) Vendors are happy to oblige and offer customized solutions to their managerial clients, but this leads to data fragmentation, where different departments and units have different versions of the truth.
Data teams need to transform the data architecture to give more power to users. End users should decide which reporting tools to use. The role of the data team is to support the infrastructure so users can analyze the data. Cloud databases should work with local company databases.
The data fabric — the matrix of information within the company — must be accessible to everyone, and one way to standardize it is through data modeling. Everyone in the company should use the same numbers and speak the same language. Consistency is so important. Scientists and engineers might understand the data architecture of a company, but not everyone else will. Data fabric makes the information available to all.
Chapter 7
During World War II, a group of mathematicians and statisticians had secret meetings in New York where they analyzed military data and made recommendations to Washington (which were frequently followed). One of the guys on the team, Abraham Wald, had been asked by the Air Force to design armor for airplanes. Data from returning planes showed that most of the bullet holes were located around the tail gunner and the wings, so people thought these were the areas that should wear the armor. (The armor was heavy so they couldn’t just slap it on the whole plane. They had to be selective.) But Abraham Wald pointed out that the planes that had been shot in the wings were the planes that lived to tell the tale. The authors tell this story to illustrate the importance of avoiding data bias.
There are many potential pitfalls, many types of data biases, that can prevent you from understanding data:
- Survivorship bias — Any time you cut data from your analysis, you risk distorted results. Correlation is not causation; just because two things seem to go together doesn’t mean that the one caused the other.
- Anchoring bias — This occurs when someone suggests a value to you and it affects your own estimate. For example, if I ask whether Gandhi was over 114 when he died, your answer would probably be different than if I asked whether he was over 35.
- Availability bias — If you see something happen or hear about how it happened from someone you know, it will seem like a lot more common an occurrence.
You can have illusions of validity and believe that gathering more data will help predict the future, but there are lots of ways you can fail to interpret the data correctly. Be careful.
New Facebook employees attend a two-week data camp to become more data literate. This gives everyone a common background for discussing problems and opportunities. They learn about available tools and data sets. They also get the opportunity to work on projects to expand their knowledge. Data teams can do a lot to meet with employees and increase data literacy across the company. Addressing company culture in this manner is an important part of their job.
Chapter 8
Descriptive analytics ask what happened; diagnostic analytics asks why. (Dashboards, which are sort of maligned throughout this book, are the interfaces of descriptive analytics.)
Descriptive and diagnostic analytics look at the past, while predictive and prescriptive analytics are about the future. Predictive analytics use historical data to predict future outcomes. Analysts can pose hypothetical “what if” questions to decide which path to take. Prescriptive analytics recommend the course of action based on the data. This requires lots of data and sophisticated analytics.
The Data Sophistication Journey is a model developed by Gartner, a marketing research agency. Data Sophistication maps a team’s evolution from descriptive to diagnostic analytics and from predictive to prescriptive analytics. But Gartner misses something between diagnostic and predictive analytics: exploratory analytics. This helps us find a hypothesis; this asks “why?” Confirmative analytics is used to determine if a hypothesis is true.
Data is only useful if you can act on it. Collecting data for no real reason serves no real purpose. On the other hand, you don’t always know what metrics will be actionable until after you’ve done an analysis. It’s good to have a balance.
Certain metrics are tried and true. The lifetime value of a customer (LTV) is an estimate of the total gross profit to be made from a customer over time. The cost of customer acquisition (CAC) is the total of all sales and marketing expenses averaged for one customer. The LTV/ CAC ratio indicates how efficiently a company pulls in revenue. But sometimes new metrics can be tailored to fit a situation, and creating new metrics can uncover new opportunities. The mMedia site Upworthy tracks various metrics to assess which factors make their content more popular, but they needed more information, so they invented a whole new metric to measure actual user attention (i.e., not accounting for those moments in which a web page open but the reader went to feed the cat). .
Design an experiment. Determine actionability. The data should relate to actual decisions that can be made. Bookend the expected results. Determine ahead of time the parameters of the experiment. Design the experiment. Develop a hypothesis. Decide on several different data points. Calculate the p-value. The p-value is the probability that the hypothesis is incorrect. Instructions for this calculation are provided. Plan to run the experiment. Figure out how long it will take. How many samples you need. Who will do the work, and how it will be structured. Don’t forget to include a control group to check results against. Run the experiment. Analyze the results. Compare them to the control group.
Chapter 9
New York City made a bunch of data available to the public. One fellow, Ben Wellington, began analyzing the data and reported about it on his blog, includinged things like mapping bicycle accidents in the city. He became very popular, and he credits his success to his storytelling abilities.
Wellington’s lessons include the importance of making data relatable. Turn it into stories. (I tell my team this all the time!) Some people think data is kind of boring, and it isn’t enough by itself to inspire them. By making it a story, you give it emotional appeal. It is particularly important to be able to tell your story when you’re courting investors. Entrepreneurs pitching startups to investors need to show that they have identified a new opportunity — demonstrate urgency.
A standard method of communicating data is through presentations. Start by defining the goal of the presentation. What are you trying to explain? Are you trying to convince someone of something? Are you trying to sell something? Evaluate the intended audience. Investors are particularly interested in risk, so discussing these points shows that you understand the investor’s perspective. (There are many kinds of risk, see sidebar.) It’s important to develop the story arc. With your knowledge of the investors’ hopes and worries, create a storyline that addresses those concerns. Aim to keep it to ten 10 slides or less.
The presentation could begin with the company purpose or mission. Describe the problem. What is wrong that your product will solve? Then offer a solution to the problem. Explain what makes this a good idea right now, and why someone didn’t do it before. A demonstration of your product would be nice, but even pictures are good. Other important information to include in your presentation: market size, your team, business model, the competition, and financials.
Communicate your vision of the opportunity, and reinforce the vision with data. Provide a solution. Explain the company’s approach. Demonstrate how the market has responded. Engagement and acquisition metrics will be helpful here. It’s important to offer a good estimate of the market size. Venture capitalists totally want to know about this. How big of a potential market are we talking here? A discussion of the financials should at minimum include revenue, gross margin, and cash flow.
When you give the presentation, people will have questions. The more data you have, the better prepared you’ll be to answer those questions.
Some different kinds of risk:
Market timing risk — Is this the right time for this enterprise?
Business model risk — Do you have the right model for your product?
Market adoption risk — Will people use your new product?
Market size risk — Is your solution big enough to make a venture capitalist happy?
Execution risk — Does your team have the right skills for the job?
Technology risk — If new technology is developed, will it be finished on schedule?
Capitalization risk — Is there enough capital to go the distance?
Platform risk — Are there external partners outside your control?
Venture management risk — Is the company open to feedback?
Financial risk — Can the company keep paying the bills?
Legal risk — Are lawsuits or other legal issues looming on the horizon?
Chapter 10: Putting it all together
Putting It All Together
There can be lots of friction in a company. Data can help reduce this friction.
People need to understand and expect data. People need to be intellectually honest; the decision-making process isn’t about ego. Let the best ideas win and don’t let politics affect the choice. A company needs well defined values. It needs the right people, it needs curious people.
The best way to arm a business for this brave new world is with data, and the thirst for data is increasingly globally. It is truly changing every industry. In the future, operationalizing data will give businesses the competitive edge they need to succeed. People will immediately have the information they need to make the best decisions.
Metrics can have a profound effect on process. They can improve the way a business operates, which can give it a competitive edge. With unified data fabric and good teams, companies are transforming their industries.
Additional Resources
Review of Winning With Data by Sloan Review
Review of Winning With Data by Hacker Noon
Review of Winning With Data by Kelly Sutton
Interview with Author of Winning With Data by Mix Panel
Buy Winning With Data on Amazon
Summary of Winning With Data by Actionable Books Free