Superforecasting: Summary and Review
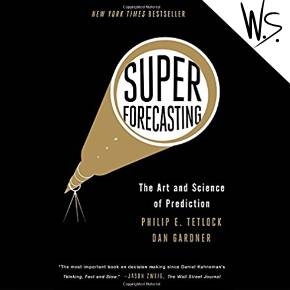
Book Review
The Intelligence Advanced Research Projects Activity (IARPA) is an intelligence agency that wanted to research the art of forecasting. They created a study where forecasters competed with one another — tournament style — to make predictions about current events.
As part of this competition, volunteers were asked to make predictions. The volunteers who consistently made the most accurate predictions — the “superforecasters” — were proven to have superior prognosticatory skills. Interestingly, the research clearly demonstrates that making accurate forecasts does not depend on any special talent — it is a learned skill.
Superforecasting: The Art and Science of Prediction is dedicated to understanding these superforecasters and exploring how an average person might become one of them. In Superforecasting, Philip Tetlock and Dan Gardner tease out a number of important qualities of superforecasters:
- Philosophic Outlook = Cautious, Humble, Nondeterministic
- Thinking Style = Open-Minded, Intelligent and Curious, Reflective, Numerate
- Forecasting Style = Pragmatic, Analytical, Dragonfly-Eyed, Probabilistic, Thoughtful Updaters, Intuitive Psychologist
- Work Ethic = Growth Mindset, Grit
Tetlock is a thorough, careful thinker, and he builds his argument slowly, block by block. He rarely telegraphs his intent, which can be frustrating for the reader who is waiting for him to reach his point. One of the results of this is that the book gets off to a very slow start. It isn’t until a third of the way through the book that Tetlock gets to the meat of his argument, and all that while, the reader isn’t entirely sure in what direction he is heading. Once he hits his stride, however, Tetlock has some interesting things to tell us about his subject, and the tone throughout the book is thoughtful and serious while remaining accessible. An advanced degree is not required to understand the ideas presented here.
Many of the ideas presented here could be useful to the head of a company, government department, or other organization. Although it isn’t explicitly spelled out, there is nothing to stop such a person from modifying these methods to suit their own needs. There would be considerable work involved in such a project, however. It would be much easier if there were a guide.
This book is not that guide. While it points the way, it is not a manual. It follows that there is room for more to be written on this subject, and one hopes that Tetlock and his cohorts will continue to explore it.
Summary
Summary note: The reader will immediately notice that there is no introduction or preface. Besides the table of contents, there is a poignant (if enigmatic) dedication and then it’s straight to business with the main body of text. The appendix includes Ten Commandments for Aspiring Superforecasters that contains practical, if elementary, advice such as, “Strike the right balance between under- and overreacting to evidence.” The scarcity of front matter will be welcome to those who skip past such material anyway when it is included. Those who desire more context will appreciate the notes with references.
Chapter 1
While some people are better at predictions than others, about 2% are superforecasters. Forecasting is a learned skill, and you can learn how to do it yourself in this book.
All sorts of people make predictions on TV and other media, but their accuracy is never actually measured. They aren’t on TV because they’re good at predicting; they are on TV because they’re good at telling a story, they are interesting, they are entertaining. The real reasons for making forecasts are not always to predict the future. Sometimes forecasts are just supposed to entertain, to persuade, or to reassure people that everything is OK. Usually these various goals go unstated.
Superforecasting discusses research showing that most experts are about as accurate in making predictions as a chimpanzee is at throwing darts at a target. (This is an apparently well-known case study that he returns to later in the book, providing much more context in Chapter 3.) The important thing that this chimpanzee study showed, however, is that while most experts weren’t very accurate in their prognostications, some were, at least with short-range analysis — the longer out, the less accurate predictions were. Predictions three to five years out approach the accuracy of the infamous dart-throwing monkey.
The Arab Spring, when revolution and change erupted across the Arab world, began with the protest of a single man, and no one could have guessed what would come of it. Scientists used to think that reality functioned with such clock-work precision that, once we understood how it worked, we’d be able to predict everything. This idea was disrupted by Edward Lorenz and the notion that a butterfly flapping its wings in Brazil could set off a tornado in Texas: chaos theory. If there is chaos, then there’s going to be unpredictability. Predictability and unpredictability are both things. One doesn’t trump the other; it’s a mix.
There is a lot we can predict, however. We can predict routine events, but even those can be dislocated by anomalies. The longer out, the harder it is to predict, but there are exceptions even to that. And while the future will likely see more of a computer-human mix in forecasting, humans are still front and center in this mix. To make good predictions you need good algorithms, but you should accept that you probably won’t always have them.
The Good Judgement Project established that some people do make good predictions. These people use specific techniques and have their own unique ways of thinking and of looking at the world. Whether or not you are one of those, following the techniques in this book will result in measurable improvement in forecasting. In this game, even small improvements are often significant over time.
Chapter 2
Tetlock begins here with a history of medicine, emphasizing how there was no methodical testing for a very long time. Good science requires healthy skepticism, and the medical profession didn’t start using randomized trials until after World War II. Instead of science, decisions were generally made based on tradition and on authority. Often enough, experts trust their own abilities and judgement without doing any research. For example, Margaret Thatcher’s government supported a policy of incarcerating young offenders in Spartan conditions. Did it work? They didn’t do any studies; they assumed their intuition knew best.
Psychologists say our mental worlds are divided into two domains. System 2 is our conscious life. It includes everything we think about. System 1 is the world of automatic reactions, autopilot functioning. The numbering is intentional — System 1 comes first. It’s always running in the background. Mulling over problems might bring us more accurate analysis, but it isn’t always practical. In the Neolithic world, people sometimes had to react quickly. System 1 is very useful. But the dichotomy between impulse and analysis is false. It’s not one or the other — the best strategy is to employ some of both.
People need to make sense of their world. When they don’t understand things, they usually make up reasons to explain it, often without conscious awareness that they are doing so. Scientists, however, are trained to have some self-discipline about their hunches. They look at other possible explanations for things; they consider the possibility that their hunch is wrong. It is important to entertain doubt, but this is counter to human nature. The natural thing is to grab onto the first plausible explanation and gather evidence that supports it while ignoring evidence that does not. Confirmation bias: we don’t like evidence that contradicts our beliefs. Another error in thought is the “bait and switch”: if we can’t answer a hard question we substitute it for an easier one.
An important factor in this conversation is pattern recognition. This helps us detect problems almost immediately, without having to think too long about things. While very useful, pattern recognition has its problems. People see the face of Jesus in their toast. They trust the pattern recognition too much. Pattern recognition is more helpful in some situation than in others. It’s a matter of knowing which situations and learning the cues. Without learning all the possible patterns, intuition is no better than random chance. But it’s hard to know if you have enough valid cues for intuition to make intuition productive, so it’s good to double-check before acting on your intuition, to make sure it passes the logic test.
Chapter 3
In order to evaluate forecasts for accuracy, we have to be able to understand exactly what the forecast says. This is more difficult than you might think.
A lot of things are not stated when people make forecasts, for example, when the forecaster assumes the audience knows the context, which might be fine until you pull out the forecast five years later and nobody remembers the context. Lots of forecasts don’t come with a timeframe, as it’s implied at the time of the forecast. But without a timeframe, forecasts are useless.
There are bigger obstacles to judging forecasts as well — for example, probability. If you say it is likely that something will happen, it’s a very different animal than saying something will happen. Tetlock recounts an instance where people were told there’s a “serious possibility” something would happen and then asked for their thoughts on the exact likelihood it would happen. Answers ranged from 20% to 80% probability, illustrating that sometimes people have very different ideas about what something means. The consequences can be disastrous.
Forecasters who express probability in numbers are forced to think more clearly about their own process. But the problem with numbers is that they seem very authoritative. People might think something is objective fact and not subjective opinion when an idea is expressed with numbers. The solution to this problem is for people to be better educated about it.
Another difficulty: say a meteorologist says there’s a 70% chance of rain. If it doesn’t rain, some people may think the forecast was wrong, but, in actuality, a 70% chance of rain means there’s a 30% chance of not rain. The only real way to judge the accuracy of the forecast would be rerun the weather 100 times and see how often it rains. But since we can’t do that, all we can really say is that the forecast was not disproved.
We can’t rerun history so we can’t judge an isolated forecast. What we can do, however, is look at a large number of forecasts together — look at the track record of a meteorologist. The question to ask is not, “Did it rain that one time when she said 70% chance of rain?” but, “Of all the times she said 70% chance of rain, did it rain 70% of the time?” This is calibration, and by calibrating the meteorologist’s forecasts (plotting percent correct by number of forecasts), you can identify whether she is under confident or overconfident. But in order to make these assessments, you need to have lots of data. It doesn’t work very well with rare events.
It’s also not very interesting or exciting to say that there’s a 60% chance of something happening — people want more information than that. The more decisive the forecaster, the better, and this is called resolution. The sweet spot is high resolution and calibration.
In the aforementioned chimpanzee study, experts were asked to make predictions, and results showed that the experts were no better than chimpanzees (or random chance). However, this average result hides important details. There were actually two different types of experts: the first were no better than random chance at making predictions; the second group did marginally better at making predictions than the chimpanzee did, but their results still were not stellar.
The difference between these groups was in their thinking. One group organized their thoughts around Big Ideas. Whether environmentalists, free-market fundamentalists, socialists, etc., these idealists fit the information they had into existing frameworks. To make an argument, they tended to pile up reasons for why their analyses were correct. They were very confident in their own abilities, even when they were wrong. The other group of analysts used a variety of tools to gather information. They were concerned with possibilities and probabilities more than certainties, and they were able to admit their errors. These experts beat the other group on both calibration and resolution.
The Big Idea people tend to organize all their thinking around the Big Idea, which distorts their view of the world. They can gather all the information they want; it won’t make them more accurate because they’re organizing it to fit in with the Idea. These people may seem confident, which makes others more likely to believe them. (These people do well on TV, even if they aren’t such good prognosticators.)
Net-net: It’s hard to see outside of our own perspective, so it’s good to aggregate information from many sources, and consider as many different perspectives as possible. Take the dragonfly: A dragonfly’s eyes are made up of many different lenses, all of which combine into a single image in the dragonfly’s mind. Tetlock suggests we should try to look at things like a dragonfly.
Chapter 4
When the U.S. invaded Iran in search of weapons of mass destruction, government analysts were certain that those weapons were there. But they were very wrong. It turns out that analysts didn’t know how well their analytical methods work because they didn’t track the accuracy of their work. The intelligence community was suitably alarmed by this state of affairs, and the Intelligence Advanced Research Projects Activity (IARPA) created a study designed to learn more about prognostication and determine how to measure it.
The study set up a tournament-style competition between forecasters, and, as part of the competition, members of the public were asked to make predictions. The people who consistently made the most accurate predictions were filtered out, and predictions from the best forecasters were weighted to magnify the results. The study found that a small minority of people were very good at forecasting; they were more accurate than most professional guessers of the future.
People don’t understand randomness. In one Yale study, people were asked to call the results of a coin toss and were then told if they were right or not. Out of 30 tosses, they were told that they were right 15 times and wrong 15 times, but the results were rigged. Some people had a flush of correct tosses early on, and these people were the most likely to have the impression that they had some sort of talent at calling coin tosses. This was false, obviously, since no skill is involved in a coin toss.
There are all sorts of logical fallacies. For example, if a TV show makes a big deal about some prognosticator because he was right on one specific occasion, it doesn’t mean anything because anyone could accidentally be right once. That’s just random odds.
Luck always plays a role, and no one, no matter how good, is infallible. But there are people who are better at forecasting the future than others. These superforecasters don’t just happen to be lucky; they have real skill. While good forecasters regress slowly over time (regression to the means = things tend back to the average), the really good forecasters barely regress at all. Year after year, there are people who maintain superforecaster status, and this wouldn’t be possible if it were just a matter of random luck.
Chapter 5
A fermi estimation is a good way to start an analysis. This involves breaking down a problem into smaller components and figuring out what you can reasonably estimate. From there, everything left is what you don’t know and you should similarly try to break down these things into as small a category as possible. This estimation process results in much more accurate estimates.
When trying to discover how common a thing is, you should start by trying to find the outside limits of the thing being considered. For example, in estimating the number of two adult and one child households that have a pet dog, you would start with the number of households that have pets and work inward from there. Often, people rush through this part, but the outside limit is important because humans tend to pay attention to the first number they have and adjust from there. The starting number, then, is an anchor, and it will keep you from drifting off into the realm of the impossible. To determine the inside limit, develop some hypotheses about the problem. Then, research the possibilities of each hypothesis.
Part of the process of synthesizing the two views is to search for outside opinions. You can also look to yourself for different perspectives. For example, assume that your first conclusion is wrong, and search for the reasons why. You can also try setting the work aside for a week or so — when you come back to it with fresh eyes, it will look different. Or change perspective by changing the wording of something; coming at it from a different angle, rephrasing the question. Forecasters need to be open-minded. They need to have curiosity, and they have to go where the evidence leads them, even when it contradicts their pet theories. Forecasters need dragonfly eyes: the ability to see many perspectives at once.
Chapter 6
If you get a bunch of people together and ask them all to predict something, they come back with a wide assortment of answers. Is that a bad thing? No, it shows that they are not engaged in groupthink; they are each using their own minds. Often, if you find an average of all their estimates, you’re going to have a pretty good approximation of the truth. This is “the wisdom of the crowd.”
The idea of probability is a modern construct which many people don’t understand. Our instincts see a simple world: a world of yes, no and maybe. (As our species evolved, we usually didn’t need more settings than that. Is something a threat? Yes- react! No- relax. Maybe- stay alert.) Probability is frustrating for people, because the human mind has a tendency to simplify everything back into the yes/no/maybe paradigm. For example, if there was a 75% chance of rain and by the end of the day it didn’t rain, that doesn’t mean the forecast was wrong — 75% chance of rain also means a 25% chance that it will not rain. But people want to know ‘yes it will rain’ or ‘no it won’t rain,’ and the best many can do with a weather forecast is to classify it as a ‘maybe.’ Superforecasters, however, usually think probabilistically, and those who wish to join their ranks need to set aside the yes/no/maybe thought pattern and learn to think in this manner.
People also like certainty, but there is always some uncertainty. This, in and of itself, is something to analyze, and there are two kinds of uncertainty: 1) You can be uncertain about things that are knowable and 2) you can be uncertain about things that are unknowable. When there’s uncertainty about unknowable things, it’s usually better to be cautious and keep predictions in the 35–65% range. In the IARPA study, estimates of 50% were the least accurate because that number was used to express uncertainty. In other words, when people say there is a 50–50% chance, this is just a fancy way of saying maybe. Good forecasters, however, tend to be very granular, having drilled down into so many details. Granularity can increase the accuracy of a prediction.
People look for meaning, particularly in times of tragedy. They look for “Why?” Some look to religion. Sometimes, when something happens, people say it was meant to happen. What are the odds, they ask, that we would have met each other on that day? But no matter how improbable, you had to be somewhere on that day, and you could have just as easily met someone else instead. Scientists don’t ask, “Why?” they ask, “How?” Superforecasters do not believe in fate.
Chapter 7
There’s no simple paint-by-number method for good forecasts. There are, however, actions that are usually helpful:
- Break the question down into smaller components.
- Identify the known and the unknown.
- Look closely at all of your assumptions.
- Consider the outside view, and frame the problem not as a unique thing but as a variant in a wider class of phenomena.
- Then, look at what it is that makes it unique; look at how your opinions on it are the same or different from other people’s viewpoints.
- Taking in all this information with your dragonfly eyes, construct a unified vision of it; describe your judgement about it as clearly and concisely as you can, being as granular as you can be.
Once a prediction is made, the work isn’t over. Predictions should be updated any time there is additional information, and superforecasters update their predictions more often than other forecasters do. These updated forecasts tend to be more accurate, because the forecaster who is updating more often is likely to be better informed.
It is tricky to update a forecast — one can underreact and one can overreact. Often, when we are confronted with new information, we want to stick to our beliefs regardless of the new evidence. People’s opinions about things can actually be more about their own self-identity than any other thing. Also, the more people who have an emotional investment in something the harder it is to admit one was wrong. Another challenge: once people publicly take a stance on something, it’s hard to get them to change their opinion. But you need to be able to change your opinion when the facts change.
It is also tricky to distinguish important from irrelevant information. Sometimes people think something is important but it’s not, and irrelevant information can confuse and trigger biases. When one doesn’t feel committed to the results, they can overreact; when they are really attached, they can underreact.
The trick is to update a forecast frequently, but, in most cases, make only small adjustments. Sometimes, of course, you need to make a dramatic change. If you are really far off target, incremental change won’t cut it.
Chapter 8
Some people think that they are what they are and that they can’t change and grow. These aren’t the people who change and grow. Because they think they can’t do it, they never try. It becomes a self-fulfilling prophecy. These people have fixed mindsets. Superforecasters have growth mindsets.
John Keynes, the famous economist, became very good at investing on the stock market. He carefully evaluated all of his failures and systematically improved his performance. He became very successful.
In order to succeed, we must try. In order to improve, we must try, fail, analyze, adjust, and try again. We learn by doing. We improve by repetition. This is true of absolutely every skill. Learning to forecast is the same way. You don’t learn it solely by reading a book. You must do it. Tetlock also explains that if you get really good at forecasting in one context — weather — it won’t translate so well to a different context — global politics. You need to apply yourself, practicing and practicing for each context.
And you have to be OK with being wrong sometimes. Making mistakes is part of the learning process. To learn from failure, we must know that we failed. So practice needs to be followed up with feedback. Without feedback, people can assume they’re doing well and become overconfident. Without feedback, people will keep thinking inaccurately about their own performance. Feedback should be immediately after the event, when everything is still fresh in our minds. Otherwise, hindsight bias sets in — once we know the outcome of something, it influences our memory of events.
Postmortems, then, are very important. Thoroughly deconstruct your forecast after the case. What did you get right? What did you get wrong? Why? And understand that just because the thing you predicted came to pass, it doesn’t necessarily follow that your process was solid — it could have just been coincidence. It is human nature to want to take credit for correct forecasts and minimize the element of chance, but dispassionate analysis will help you to improve.
Perpetual beta means continuous analysis and improvement. Maintaining a state of perpetual beta is way, way more important than intelligence. In fact, of all the qualities common to superforecasters, the quality that does the best job of predicting who will become a superforecaster is that of perpetual beta. In this context, grit and tenacity are important qualities.
Chapter 9
The Bay of Pigs Invasion was poorly planned and executed. The Kennedy administration lost credibility, but things changed during the Cuban Missile Crisis. It was pretty much the same team that handled both events.
After Bay of Pigs, Kennedy launched an investigation to figure out what went wrong. The decision-making process was identified as the problem. The team members were victims of groupthink, which happens because people want to get along with each other. Sometimes, they will subconsciously adjust their beliefs to go along with the team. Whole groups of people can drift away from any rational moorings in this way.
The Kennedy team developed a new, skeptical method and they began to question their assumptions. Sometimes Kennedy would purposely leave the room to give the team space to throw around ideas without the boss around. This was really valuable. Ultimately, when the Cuban Missile Crisis came about, they were able to generate all sorts of alternative solutions. Their improved method may well have spared the world a nuclear war.
This demonstrates that it’s possible for a group to change their decision-making process for the better. There is no need to search for the perfect group when a motivated team can learn to change. And despite the risks of groupthink, working in a team can sharpen judgement and reach greater goals than individuals can achieve alone. Tetlock asks the question: Should forecasters work in teams, or should each work individually?
- Disadvantages: Teams can make people lazy. Let other people do the work, they tell themselves, while we loiter in the back office playing pinochle. Also, teams can be susceptible to groupthink.
- Advantages: People can share information when they work in teams. They can share perspectives. With many perspectives, dragonfly eye becomes more accessible. Aggregation is so important.
To determine whether the advantages and disadvantages cancel each other out, they did a study to see if teams of forecasters worked better than individuals. The results were unambiguous: teams are clearly more accurate than people. Furthermore, when superforecasters were put together in teams, they out-forecasted the prediction markets.
These findings, although not an automatic recipe for success, highlight the importance of good group dynamics. Teams should also be open minded; they should have a culture of sharing. Finally, diversity is exceptionally important — even more so than ability. Superteams composed of diverse people with different perspectives have more information to go on.
Chapter 10
Superteams operate best with flat, nonhierarchical structures. But businesses and governments — who need forecasters to help them with their decisions — very much are hierarchical. How can these fit together? It is possible to foster a flat, flexible structure in a hierarchical organization? Interestingly, Tetlock uses the Wehrmacht as an example. (He does so to illustrate the need to separate feelings and biases — for example, reactions to or discomfort with the Wehrmacht as a model — so they don’t influence predictions.)
In the nineteenth century, the Prussian army achieved victory over their neighbors. The Prussians understood that uncertainty was an important part of reality, and Prussian leaders gave a lot of thought to uncertainty. It was important to realize that circumstances can change very fast. Because of this emphasis on uncertainty, officers were trained to be flexible so they could handle situations as they emerged. Even soldiers were encouraged to question authority when appropriate. This principle was known as “auftragstaktik”, literally, mission command. In war, decisions needed to be made locally to respond to changing situations. Commanders told subordinates what the goal was, but not how to achieve it. Even the lowliest soldier was expected to act with autonomy.
The Nazis inherited this army: the Wehrmacht. The Wehrmacht were very successful for a long time. Ultimately, however, they were overwhelmed by superior forces. Their defeat was hastened by mistakes, including Hitler’s autocratic leadership which violated the principles of auftragstaktik.
Comparatively, in the US Army, subordinates were never allowed to question their superiors. They received lengthy, detailed orders that spelled out every action they were to take. There were few exceptions. The US Army didn’t learn the auftragstaktik lesson until the 1980s. They arguably still have a way to go, but they’ve become much more decentralized since then. When the US invaded Iraq, General Petraeus was empowered to respond to the circumstances he found on the ground and was able to minimize resistance. He stressed the importance for his people to learn to think flexibly and deal with things as they came up; the importance of looking at things from different perspectives.
Chapter 11
A big trap that we encounter is to think that what you see is all there is. We can all make mistakes and forget to double check our assumptions. And often, we don’t pay enough attention to the scope of a question. For example, if you asked someone, “Will the Assad reign in Syria fall this year?” their answer will reflect whether they think the Assad reign will ever fall. They are insensitive to scope.
Superforecasters demonstrate better — not perfect, but way better — scope sensitivity than regular forecasters. They are using System 2 to check on System 1 so regularly that it becomes automatic. In this way, System 2 becomes a part of System 1.
In a book about forecasting, Tetlock naturally must discuss Nassim Taleb’s black swan. Originally, all swans in Europe were white. If you asked an Englishman to imagine a really weird swan he’d probably think of one that was abnormally sized or one with a funny beak or something. But all the different swans in his imagination would likely be white, because he’d never seen a swan of a different color. He couldn’t imagine one even when he was trying to think of a different kind of swan. Then, in the 17th Century, British boats travel to Australia and back, bringing some souvenirs and oddities with them, including a black swan. The Englishman is seeing something he couldn’t have even imagined previously. Mind = blown.
The whole theory about black swans as developed by Nassim Taleb endangers Tetlock’s theory, so he deconstructs it for readers. How do you precisely define “black swan”? If you mean something that was previously inconceivable, that is extremely rare, indeed. Or maybe you could water it down to “highly improbably consequential events.” It’s very hard to get data on highly improbably events — by their nature, they don’t happen often. The Good Judgment Project hasn’t run long enough to collect enough data on it.
And if you look closely at supposed black swan events, they were mostly, but not precisely, predictable. Forecasts are less accurate the further in the future they predict. A prediction’s accuracy decreases with time, until about five years out when it comes equal with chance. So, long-term forecasts aren’t viable. Nevertheless, all sorts of companies and institutions make long-range forecasts. Sometimes they are needed, so that long-range plans may be made. In these cases, the best thing to do is to prepare for surprise. Plan resilience and adaptability; imagine different scenarios where unlikely things happen and decide how you’d respond.
Chapter 12
Sometimes people are hostile to forecasters who predict things they don’t like. Conversely, they may be especially friendly with those who prognosticate what they want to hear. Sometimes politics are more powerful than predictions. Sometimes people use forecasts to defend their interests or those of their tribe, and, in such situations, accuracy often takes a back seat. Some people cling to the status quo because they are afraid of change, but they can be persuaded through good research. (Tetlock leverages inspiring examples of people who used evidence and analytics to sway people’s opinions and change society.)
For many reasons, good forecasting is so important. It can make the difference between success and failure, between peace and war. And keeping score and tracking results is the best way to evaluate predictions. This will help forecasters improve. This will also help us hold people accountable for making vague predictions that can’t be measured. We need to get serious about keeping score.
The Brier score — measuring the difference between a prediction and the actual result — is pretty good, but, like anything, there’s always room for improvement. Fortunately, the rise of information technology has accelerated our abilities to count and to test. Athletes, for example, have been able to make remarkable improvements in their performances because of the systematic search for evidence-based solutions.
However, while numbers are lovely things, they are only tools, after all. We can’t assign metrics to things that are essentially uncountable. Sometimes the really important questions are the hardest to score. Sometimes you have to look at a complex situation and break it down into smaller questions. A better methodology for this needs to be developed.